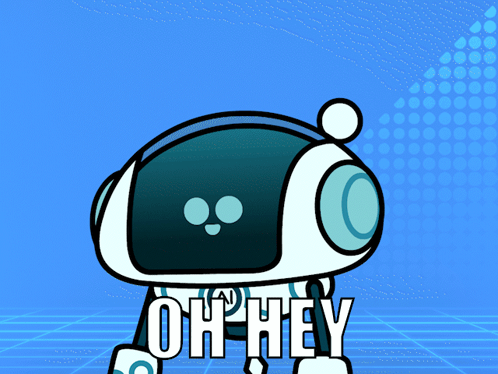
Introduction:
Artificial intelligence technology known as "generative AI" is capable of producing text, images, audio, and synthetic data, among other kinds of content. The ease of use of new user interfaces that enable the creation of excellent text, pictures, and movies in a matter of seconds has been the driving force behind the recent excitement surrounding generative AI.
Transformers and the revolutionary language models they made possible are two other recent developments that will be covered in more detail below and have been essential in the mainstreaming of generative AI. Thanks to a sort of machine learning called transformers, scientists can now train ever-larger models without having to classify all of the data beforehand. Thus, billions of text pages might be used to train new models, producing responses with greater nuance. Transformers also opened the door to a novel concept known as attention, which allowed models to follow word relationships not just inside sentences but also throughout pages, chapters, and books. Not only that, but Transformers could analyse code, proteins, molecules, and DNA with their ability to track connections.
With the speed at which large language models (LLMs) are developing, i.e., models with billions or even trillions of parameters, generative AI models are now able to compose captivating text, produce photorealistic graphics, and even make reasonably funny sitcoms on the spot. Furthermore, teams are now able tFo produce text, graphics, and video material thanks to advancements in multimodal AI. Tools like Dall-E that automatically produce images from text descriptions or text captions from photographs are based on this.
How does generative AI work?
A prompt, which can be any input that the AI system can handle, such as a word, image, video, design, musical notation, or other type of input, is the first step in the generative AI process. After that, different AI algorithms respond to the instruction by returning fresh content. Essays, problem-solving techniques, and lifelike fakes made from images or audio of real people can all be considered content.
In the early days of generative AI, data submission required the use of an API or other laborious procedures. The developers needed to learn how to use specialised tools and write programs in languages like Python.
How does generative AI do?
These days, generative AI pioneers are creating improved user interfaces that enable you to express a request in simple terms. Following an initial response, you can further tailor the outcomes by providing input regarding the tone, style, and other aspects you would like the generated content to encompass.
To represent and analyse content, generative AI models mix several AI techniques. To produce text, for instance, different natural language processing methods convert raw characters (such as letters, punctuation, and words) into sentences, entities, and actions. These are then represented as vectors using a variety of encoding techniques. In a similar way, vectors are used to express different visual aspects from photographs. A word of caution: the training data may contain bigotry, prejudice, deceit, and puffery that these techniques can also encode.
Developers use a specific neural network to create new information in response to a prompt or question once they have decided on a representation of the world. Neural networks comprising a decoder and an encoder, or variational autoencoders (VAEs), are among the techniques that can be used to create artificial intelligence training data, realistic human faces, or even individualised human effigies.
Recent developments in transformers, such Google's Bidirectional Encoder Representations from Transformers (BERT), OpenAI's GPT, and Google AlphaFold, have also led to the development of neural networks that are capable of producing new content in addition to encoding text, images, and proteins.
What are ChatGPT, Bard, and Dall-E?
Popular generative AI interfaces are ChatGPT, Dall-E, and Bard.
Dall-E: Dall-E is an example of a multimodal AI application that recognizes links across different media, such as vision, text, and audio. It was trained on a large data set of photographs and the text descriptions that go with them. Here, it links the meaning of the words to the visual components. In 2021, OpenAI's GPT implementation was used in its construction. In 2022, a more competent version, Dall-E 2, was released. With the help of cues from the user, it allows users to create graphics in various styles.
ChatGPT: OpenAI's GPT-3.5 implementation served as the foundation for the AI-powered chatbot that swept the globe in November 2022. Through a chat interface with interactive feedback, OpenAI has made it possible to communicate and improve text responses. GPT's previous iterations could only be accessed through an API. Released on March 14, 2023, GPT-4. ChatGPT simulates a real conversation by including the history of its communication with a user into its output. Microsoft announced a large new investment into OpenAI and included a version of GPT into its Bing search engine following the new GPT interface's phenomenal popularity.
Bard: When it came to developing transformative AI methods for analysing language, proteins, and other kinds of content, Google was a trailblazer as well. For researchers, it made some of these models publicly available. It never did, however, make these models' public interface available. Due to Microsoft's decision to integrate GPT into Bing, Google hurried to launch Google Bard, a chatbot for the general public that is based on a streamlined variant of its LaMDA family of large language models. After Bard's hurried introduction, Google's stock price took a big hit when the language model mispronounced the Webb telescope's discovery of a planet in a different solar system as the first. In the meanwhile, inconsistent behaviour and erroneous results cost Microsoft and ChatGPT implementations in their initial forays.
What applications does generative AI have?
Almost any type of material may be produced with generative AI in a variety of use cases. Modern innovations such as GPT, which can be adjusted for many uses, are making technology more approachable for people of all stripes. The following are a few examples of generative AI's applications:
- Using chatbots to assist with technical support and customer service.
- Use deepfakes to imitate particular persons or groups of people.
- Enhancing the dubbing of films and instructional materials in several languages.
- Composing term papers, resumes, dating profiles, and email replies.
- Producing work in a specific style that is photorealistic.
- Enhancing the videos that show off products.
- Offering novel medication combinations for testing.
- Creating tangible goods and structures.
- Improving the designs of new chips.
What advantages does generative AI offer?
Generative AI has broad applications in numerous business domains. It can automatically generate new material and facilitate the interpretation and understanding of already-existing content. Developers are investigating how generative AI may enhance current processes, with the goal of completely changing workflows to leverage the technology. The following are some possible advantages of applying generative AI:
- Automating the laborious task of content creation by hand.
- Lowering the time it takes to reply to emails.
- Enhancing the answer to particular technical inquiries.
- Making people look as authentic as possible.
- Assembling complicated data into a logical story.
- Streamlining the process of producing material in a specific manner.
What are generative AI's limitations?
The numerous limits of generative AI are eloquently illustrated by early implementations. distinct techniques used to implement distinct use cases give rise to some of the issues that generative AI brings. A synopsis of a complicated subject, for instance, is simpler to read than an explanation with multiple references for important topics. Nevertheless, the user's capacity to verify the accuracy of the information is compromised by the summary's readability.
The following are some restrictions to take into account when developing or utilising a generative AI application:
- It doesn't always reveal the content's original source.
- Evaluating original sources for bias might be difficult.
- Content that sounds realistic can make it more difficult to spot false information.
- It can be challenging to figure out how to adjust for novel situations.
- Outcomes may mask prejudice, bigotry, and hatred.
What worries people about generative AI?
Concerns of a variety are also being stoked by the emergence of creative AI. These have to do with the calibre of the output, the possibility of abuse and exploitation, and the ability to upend established corporate structures. Here are a few examples of the particular kinds of challenging problems that the status of generative AI currently poses:
- It may offer false and deceptive information.
- Without knowledge of the information's origin and source, trust is more difficult to establish.
- It may encourage novel forms of plagiarism that disregard the rights of original content creators and artists.
- It might upend current business structures that rely on advertising and search engine optimization.
- It facilitates the production of false news.
Industry use cases for generative AI
Because of their substantial impact on a wide range of sectors and use cases, new generative AI technologies have occasionally been compared to general-purpose technologies like steam power, electricity, and computing. It's important to remember that, unlike earlier general-purpose technologies, instead of just speeding up small bits of current processes, it frequently took decades for people to figure out how to best structure workflows to take advantage of the new method. The following are some potential effects of generative AI applications on various industries:
- In order to create more effective fraud detection systems, finance can monitor transactions within the context of an individual's past.
- Generative AI can be used by law companies to create and understand contracts, evaluate evidence, and formulate arguments.
- By combining data from cameras, X-rays, and other metrics, manufacturers can utilise generative AI to more precisely and cost-effectively identify problematic parts and their underlying causes.
- Generative AI can help media and film firms create material more affordably and translate it into other languages using the actors' voices.
- Generative AI can help the medical sector find promising drug candidates more quickly.
- Generative AI can help architectural firms create and modify prototypes more quickly.
- Generative AI can be used by gaming businesses to create game levels and content.
The best ways to apply generative AI
Depending on the modalities, methodology, and intended goals, there are several best practices for applying generative AI. Having said that, when utilising generative AI, it's critical to take into account crucial elements like accuracy, transparency, and tool simplicity. The following procedures aid in achieving these elements:
- Give every piece of generative AI content a clear title for viewers and users.
- Verify the content's accuracy using primary sources where necessary.
- Think about the ways that bias could be included into AI outcomes.
- Use additional tools to verify the accuracy of AI-generated material and code.
- Discover the benefits and drawbacks of any generative AI technology.
- Learn about typical result failure modes and devise workarounds for them.
Conclusion:
The remarkable complexity and user-friendliness of ChatGPT encouraged generative AI to become widely used. Undoubtedly, the rapid uptake of generative AI applications has also highlighted certain challenges in implementing this technology in a responsible and safe manner. However, research into more advanced instruments for identifying text, photos, and video generated by AI has been spurred by these early implementation problems.
Indeed, a plethora of training programs catering to various skill levels have been made possible by the growing popularity of generative AI technologies like ChatGPT, Midjourney, Stable Diffusion, and Bard. The goal of many is to assist developers in creating AI applications. Others concentrate more on business users who want to implement the new technology throughout the company.
navan.ai has a no-code platform - nstudio.navan.ai where users can build computer vision models within minutes without any coding. Developers can sign up for free on nstudio.navan.ai
Want to add Vision AI machine vision to your business? Reach us on https://navan.ai/contact-us for a free consultation.