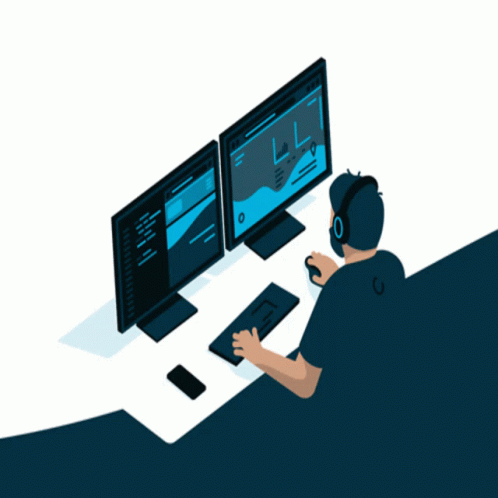
Introduction:
It is now more important than ever to guarantee the operation, dependability, and general quality of software programs. By applying methodical procedures and approaches to assess and improve software quality, quality assurance is essential to reaching these goals. With technology developing at a breakneck speed, fresh and creative ideas are being developed to address the problems associated with software quality. Using generative artificial intelligence (Generative AI) is one such strategy.
Activities aimed at ensuring software products meet or surpass quality standards are referred to as quality assurance. The capacity of software quality to improve an application's dependability, efficiency, usability, and security makes it crucial. The aim of quality assurance (QA) specialists is to find software flaws and vulnerabilities so that risks may be reduced and end users can be satisfied. They achieve this by putting strict testing procedures into place and performing in-depth code reviews.
There has been a lot of interest in generative AI. Generative AI uses machine learning techniques to produce novel and creative outputs based on patterns and data it has been trained on, in contrast to classic AI systems that depend on explicit rules and human-programmed instructions.
Generative AI can be used in the quality assurance environment to automate and optimise certain QA process steps. Pattern recognition, anomaly detection, and potential problem prediction that could affect software quality are all capabilities of generative AI models. Early defect discovery is made possible by this proactive strategy, which enables QA and development teams to take corrective action and raise the overall standard of the program. Furthermore, synthetic test data generation and test case generation automation can be facilitated by Generative AI.
The incorporation of Generative AI into software development, as technology develops, has promise for optimising quality assurance endeavours and facilitating the creation of software applications that are more resilient, dependable, and intuitive.
Comprehending Generative Artificial Intelligence for Software Quality Assurance
The notion of generative artificial intelligence
The field of artificial intelligence has undergone a paradigm change with the introduction of generative AI, which emphasises machines' capacity to create unique material instead of only adhering to preset guidelines. With this method, machines can learn from large datasets, spot trends, and produce results based on that understanding.
Deep learning and neural networks are two methods used by generative AI models to comprehend the underlying structure and properties of the data they are trained on. These models are able to produce new instances that are similar to the training data, but with distinctive variants and imaginative components, by examining patterns, correlations, and dependencies. Because of its creative ability, generative AI is a potent tool for software quality assurance among other fields.
Generative AI's Place in Software Testing
The creation of test cases is an essential component of software testing since it impacts the process's efficiency and breadth of coverage. Software testers have historically created test cases by hand. This can be done manually, which can be laborious and prone to error, or with the aid of test automation tools. Nevertheless, test case generation can be done more effectively and automatically with generative AI approaches, which enhances testing process speed and quality.
Improving the Generation of Test Cases
In order to understand the patterns and logic that underlie a software system, generative AI models can examine user needs, specifications, and existing software code. These models are capable of producing test cases that span a wide range of scenarios, including both expected and edge cases, by comprehending the links between inputs, outputs, and expected behaviours. In addition to lowering the amount of manual labour needed, this automated test case development expands the testing process's coverage by examining a greater variety of potential inputs and scenarios.
Recognizing Complicated Software Problems
Furthermore, generative AI is particularly good at spotting complicated software bugs that could be hard for human testers to find. Complex connections, non-linear behaviours, and interactions in software systems can result in unforeseen vulnerabilities and flaws. Large volumes of software-related data, such as code, logs, and execution traces, can be analysed by generative AI models to find hidden patterns and abnormalities. These models identify possible software problems that could otherwise go undetected by distinguishing abnormalities from expected behaviour. Early identification makes it possible for QA and development teams to quickly address important problems, which results in software that is more dependable and robust.
The advantages of generative AI
QA gains a great deal from generative AI. Because of its special abilities and methods, there are more opportunities to increase test coverage, improve issue identification, and hasten software development. The testing industry benefits from it in the following ways:
1. Enhanced Efficiency and Test Coverage
The capacity of generative AI to increase test coverage is its main advantage for software quality assurance. Generative AI models may automatically produce extensive test cases that cover a variety of scenarios and inputs by utilising algorithms and vast datasets. The effort needed is decreased while the testing process is made more comprehensive and efficient thanks to this automated test case generation.
Consider a web application that needs to be tested on many platforms, devices, and browsers. With the use of generative AI, test cases covering various combinations of platforms, devices, and browsers may be produced, providing thorough coverage without requiring a lot of human environment setup or test case generation. As a result, testing becomes more effective, bugs are found more quickly, and trust is raised.
2. Improving Bug Detection
Complex software problems that may be difficult for human testers to find can be quickly found by generative AI. Large amounts of software-related data, including code and logs, are analysed by these methods in order to find trends and deviations from typical application behaviour. Generative AI models are able to identify possible flaws, vulnerabilities early in the development process by identifying these abnormalities.
Take into consideration, for instance, an e-commerce platform that must guarantee the precision and dependability of its product suggestion system. By creating fictitious user profiles and modelling a range of purchase habits, generative AI can greatly improve testing and development of such systems.
3. Generative AI-Assisted Software Development Acceleration
By streamlining several phases of the development lifecycle, generative AI not only improves the quality assurance process but also speeds up software development. With the help of generative AI, developers can concentrate more on original thinking and creative problem-solving by automating processes like test case creation, code reworking, and even design prototyping.
For instance, generative AI can help with the autonomous generation of design prototypes in the field of software design, depending on user preferences and requirements. Generative AI models can suggest fresh and inventive design options by examining current design patterns and user feedback. This shortens the time and effort needed to develop a refined design and expedites the design iteration process.
Implementing Generative AI Presents Challenges
AI technologies to replace testers
There is still disagreement over the idea of AI completely replacing software testers. Even though generative AI can automate some steps in the testing process, software testing still greatly benefits from human expertise and intuition. AI models are trained using available data, and the calibre and variety of the training data has a significant impact on the models' efficacy. They might, however, find it difficult to handle peculiar situations or recognize problems that are unique to a given setting and need for human judgement
In addition to finding faults, software testing also entails determining usability, comprehending user expectations, and guaranteeing regulatory compliance. These elements frequently call on domain expertise, human judgement, and critical thinking. Although generative AI can improve and supplement testing, it is more likely to supplement rather than completely replace software testers in their duty.
Appropriate Use of AI
As AI technologies develop, it's critical to address ethical issues and make sure AI is used responsibly in software testing. Among the crucial factors are:
1. Fairness and Bias:
When generative AI models are trained on historical data, biases may be introduced if the data represents imbalances or biases in society. Selecting training data with care and assessing the fairness of AI-generated results are crucial.
2. Data security and privacy:
When generative AI is used, huge datasets that can include private or sensitive data are analysed. To preserve user privacy, it is essential to follow stringent privacy and data protection laws, get informed consent, and put strong security measures in place.
3. Openness and Definability:
AI models can be intricate and challenging to understand, particularly generative AI based on deep learning. Building trust and comprehending how the system generates its outputs depend on ensuring openness and explainability in AI-driven decisions.
4. Liability and Accountability:
Since AI has been used in software testing, concerns about responsibility and liability may surface when decisions made by AI have an adverse effect on users or produce unintended results. Addressing potential legal and ethical ramifications requires defining duty and establishing clear accountability mechanisms.
5. Openness and Definability:
AI models can be intricate and challenging to understand, particularly generative AI based on deep learning. Building trust and comprehending how the system generates its outputs depend on ensuring openness and explainability in AI-driven decisions.
6. Liability and Accountability:
Since AI has been used in software testing, concerns about responsibility and liability may surface when decisions made by AI have an adverse effect on users or produce unintended results. Addressing potential legal and ethical ramifications requires defining duty and establishing clear accountability mechanisms.
Apart from these particular activities, generative AI is anticipated to be employed to enhance the efficacy and efficiency of automated software testing on a broader scale. Generative AI, for instance, can be utilised to:
1. Sort test cases into priority lists:
The most likely test cases to uncover bugs can be found using generative AI. This can assist in concentrating testing efforts on the most important domains.
2. Automate upkeep of tests:
Test case maintenance can be automated with generative AI. This can guarantee that tests are updated in response to program modifications.
Conclusion:
The incorporation of generative AI approaches is the way forward for automated software testing. Promising potential for improved test data generation, intelligent test case development, adaptive testing systems, test scripting and execution automation, test optimization, and resource allocation will arise as generative AI develops.
Generative AI has a bright future in automated software testing. Generative AI is expected to advance in strength and versatility as it develops further. This will create new avenues for increasing software quality and automating software testing.
navan.ai has a no-code platform - nstudio.navan.ai where users can build computer vision models within minutes without any coding. Developers can sign up for free on nstudio.navan.ai
Want to add Vision AI machine vision to your business? Reach us on https://navan.ai/contact-us for a free consultation.