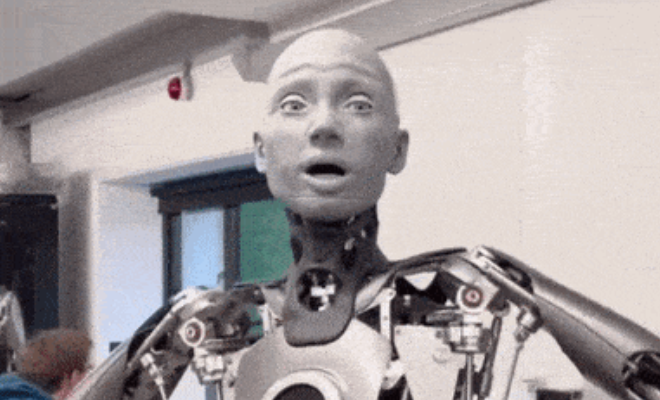
Introduction:
Imagine a future where all tasks, no matter how simple or complex, are completed more quickly, intelligently, and effectively. This is the current state of affairs. Modern technology combined with clever automation techniques has brought about previously unheard-of levels of productivity improvements.
By 2030, the market for digital workforce is projected to have grown by 22.5% year and reach $18.69 billion. With such rapid growth predicted, companies must implement worker automation.
Investigate the intelligent automation technologies that apply to your sector and methods now. Determine which areas within your company can benefit from a digital transformation in terms of increased productivity, lower expenses, and improved processes. You'll discover how digital workers & AI agents are transforming corporate procedures in this article.
An AI Digital Worker: What Is It?
Artificial intelligence (AI) digital workers are neither people nor robots. Rather than that, it's a completely new method of workplace automation. To support your marketing objectives, consider them as collections of technologies and data that can perform jobs and combine ideas.
Digital assistants with artificial intelligence should ideally be engaged team members that can support your human staff while managing regular duties. They are hired to relieve you of tedious tasks so that your employees can concentrate on strategically important, strategically engaging work that advances your company.
What are AI agents?
An artificial intelligence (AI) agent is a software application that can interact with its surroundings, interpret data, and act in response to the data in order to accomplish predetermined objectives. Artificial intelligence (AI) agents can mimic intelligent behaviour; they can be as basic as rule-based systems or as sophisticated as sophisticated machine learning models. They may require outside oversight or control and base their choices on predefined guidelines or trained models.
An advanced software program that can function autonomously without human supervision is known as an autonomous AI agent. It is not dependent on constant human input to think, act, or learn. These agents are frequently employed to improve efficiency and smooth operations across a variety of industries, including banking, healthcare, and finance.
For example:
Text responses resembling those of a person can be produced by the AI agent AutoGPT, which is capable of understanding the conversation's context and producing pertinent responses in line with it.
An intelligent virtual agent called AgentGPT was created with the purpose of interacting with clients and offering tailored advice. In response to inquiries from customers, it can comprehend natural language and deliver pertinent answers.
Characteristics of an AI agent:
1. Independence:
An artificial intelligence (AI) virtual agent can carry out activities on its own without continual human assistance or input.
2. Perception:
Using a variety of sensors, including cameras and microphones, the agent function senses and interprets the world in which they operate.
3. Reactivity:
To accomplish its objectives, an AI agent can sense its surroundings and adjust its actions accordingly.
4. Reasoning and decision-making:
AI agents are intelligent tools with the ability to reason and make decisions in order to accomplish objectives. They process information and take appropriate action by using algorithms and reasoning processes.
5. Education:
Through the use of machine, deep, and reinforcement learning components and methodologies, they can improve their performance.
6. Interaction:
AI agents are capable of several forms of communication with people or other agents, including text messaging, speech recognition, and natural language understanding and response.
Structure of an AI agent:
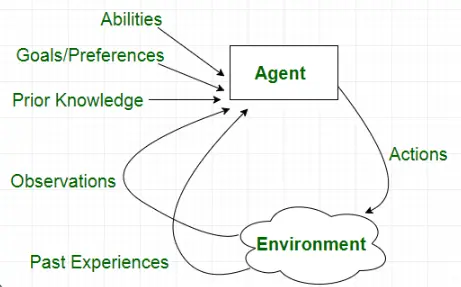
1. Environment
The realm or area in which an AI agent functions is referred to as its environment. It could be a digital location like a webpage or a physical space like a factory floor.
2. Sensors
An AI agent uses sensors as tools to sense its surroundings. These may be microphones, cameras, or any other kind of sensory input that the AI agent could employ to learn about its surroundings.
3. Actuators
An AI agent employs actuators to communicate with its surroundings. These could be computer screens, robotic arms, or any other tool the AI agent can use to modify the surroundings.
4. Decision-making mechanism
An AI agent's decision-making system is its brain. It analyses the data acquired by the sensors and uses the actuators to determine what needs to be done. The actual magic occurs in the decision-making process. AI agents make educated decisions and carry out tasks efficiently by utilising a variety of decision-making methods, including rule-based systems, expert systems, and neural networks.
5. Learning system
The AI agent can pick up knowledge from its experiences and interactions with the outside world thanks to the learning system. Over time, it employs methods including supervised learning, unsupervised learning, and reinforcement learning to enhance the AI agent's performance.
How does an AI Agent work?
Step 1: Observing the surroundings
An independent artificial intelligence agent must initially acquire environmental data. It can accomplish this by gathering data from multiple sources or by using sensors.
Step 2: Handling the incoming information
After gathering information in Step 1, the agent gets it ready for processing. This could entail putting the data in order, building a knowledge base, or developing internal representations that the agent can utilise.
Step 3: Making a choice
The agent makes a well-informed decision based on its goals and knowledge base by applying reasoning techniques like statistical analysis or logic. Applying preset guidelines or machine learning techniques may be necessary for this.
Step 4: Making plans and carrying them out
To achieve its objectives, the agent devises a strategy or a set of actions. This could entail developing a methodical plan, allocating resources as efficiently as possible, or taking into account different constraints and priorities. The agent follows through on every step in its plan to get the intended outcome. Additionally, it can take in input from the surroundings and update its knowledge base or modify its course of action based on that information.
Step 5: Acquiring Knowledge and Enhancing Performance
The agent can get knowledge from its own experiences after acting. The agent can perform better and adjust to different environments and circumstances thanks to this feedback loop.
Types of AI Agents:
1. Simple reflex agents are preprogrammed to react according to predetermined rules to particular environmental inputs.
2. Model-based reflex agents keep an internal model of their surroundings and utilise it to guide decisions are known as model-based reflex agents.
3. Goal-based agents carry out a program to accomplish particular objectives and make decisions based on assessments of the surrounding conditions.
4. Utility-based agents weigh the possible results of their decisions and select the course of action that maximises predicted utility.
5. Learning agents use machine learning techniques to make better decisions.
How do AI agents and digital workers work?
Artificial intelligence digital workers employ multiple forms of artificial intelligence to do tasks. However digital agents blend large language models, or LLMs, with generative AI, which is meant to produce new material or data (made to understand, develop, and operate with human language).
LLMs and generative AI may be familiar to you from other AI tools. Popular AI chatbot ChatGPT, which uses generative AI technology to generate responses to questions, is regarded as an LLM.
1. Machine Learning (ML):
Under your guidance, digital AI staff members can learn, adjust, and eventually perform better.
2. Natural Language Processing (NLP):
Digital workers that are proficient in language are better equipped to comprehend and translate human instructions into practical steps.
3. Robotic Process Automation (RPA):
RPA, possibly the most well-known type of AI, automates jobs that are repetitive and rule-based, such as sending emails, generating content templates, and filling out spreadsheets.
Benefits Of digital workers
1. A rise in output
Digital workers don't require breaks or holidays because they can work nonstop. As a result, work may be done more quickly and effectively, increasing productivity for your company.
2. Performance devoid of errors
Digital workers are not prone to errors like people are. They ensure precise and error-free performance by adhering to predetermined rules and algorithms. This can greatly lower expensive mistakes and raise the calibre of output.
3. Savings on costs
The cost of hiring and training human labour can be high. Conversely, digital workers don't have to pay for ongoing expenses like salaries and benefits and only need to pay small upfront costs. They are therefore an affordable option for companies trying to maximize their spending.
4- Quicker reaction times
Digital workers can respond to consumer enquiries and complaints more quickly because they can manage vast volumes of data and requests at once. By offering prompt support, this contributes to improving customer satisfaction.
5-Scalability
The need for jobs to be accomplished increases along with the growth of your firm. You can scale up or down as needed with digital workers without having to worry about scarce resources or go through a drawn-out hiring procedure.
There are several advantages to integrating digital workers into your company operations, such as higher output, error-free performance, cost savings, quicker reaction times, and scalability. Businesses can succeed more and obtain a competitive edge by using this cutting-edge technology.
How do you integrate digital workers into your company?
1. Recognize recurrent duties
Determine which jobs require a lot of time and repetition first. These duties can include everything from email management and file organisation to data entry and report creation. Your digital employees will have more time to devote to more strategic endeavours if these chores are automated.
2. Pick the appropriate tools
Choosing the appropriate hardware and software solutions is essential after determining which tasks require automation. The market is flooded with automation technologies designed expressly to meet the needs of digital workers. Seek for solutions with an intuitive user interface and simple interaction with current systems.
3. Simplify procedures
Automation involves not just taking the place of manual operations but also optimising workflows. Examine your present procedures and pinpoint any places where bottlenecks arise or where extra steps might be cut. Workflows can be made more efficient for your digital workers by making sure that tasks flow smoothly from one to the next.
4- Offer guidance and assistance
You may need to provide your digital workers with some training and support when implementing automation in the workplace. Make sure they know how to utilize the new equipment and are at ease with the modifications. Provide continuing assistance and welcome input so that any necessary corrections can be made.
5-Assess development
After automation is put into place, it's critical to routinely assess its efficacy. Monitor key performance indicators (KPIs) such saving time, mistake rates, and employee satisfaction. You can use this data to determine whether any more changes or improvements are necessary.
Problems and Challenges with integrating digital workers & AI Agents:
1. Requirements for skill sets
IT know-how particular to digital workers is needed to integrate them into an organisation. This makes it difficult to hire new staff members or retrain current ones to handle the technology required to serve these remote workers.
2-Redefining the job
Employees may need to change their responsibilities or face job redundancies as a result of the arrival of digital workers. Employees who struggle to adjust to increased duties or who fear job uncertainty may react negatively to this.
3. Security of data
Data security becomes a top priority when managing sensitive information by digital workers. It is imperative for businesses to implement strong security protocols to safeguard sensitive information from any breaches or assaults.
4-Assimilation with current systems
It can be difficult and time-consuming to smoothly integrate digital workers with current IT systems. Compatibility problems could occur and force businesses to spend money on new software or equipment.
5. Moral implications
As artificial intelligence (AI) technology develops, moral questions about the employment of digital labour arise. In order to guarantee equitable and conscientious utilisation of new technologies, concerns of data privacy, algorithmic bias, and accountability must be thoroughly examined.
6. Data bias:
When making decisions, an autonomous artificial intelligence agent program mainly depends on data. Their use of skewed data may result in unjust or discriminating conclusions.
7. Absence of accountability:
Since proactive agents are capable of making decisions without human assistance, it can be challenging to hold them responsible for their deeds.
8. Lack of transparency:
Learning agents' decision-making processes can be convoluted and opaque, making it challenging to comprehend how they reach particular conclusions.
Conclusion:
Digital workers of today are built to recall previous encounters at work and absorb new ones. They can communicate with several human personnel and operate across systems and processes. The advent of a truly hybrid workforce, wherein people perform high-purpose work assisted by digital workers, could be accelerated by these skills. With this mixed workforce, the method of completion will be more important than the location of work.
navan.ai has a no-code platform - nstudio.navan.ai where users can build computer vision models within minutes without any coding. Developers can sign up for free on nstudio.navan.ai
Want to add Vision AI machine vision to your business? Reach us on https://navan.ai/contact-us for a free consultation.